- 1Department of Neurology, The First Affiliated Hospital of Chongqing Medical University, Chongqing, China
- 2NHC Key Laboratory of Diagnosis and Treatment on Brain Functional Diseases, The First Affiliated Hospital of Chongqing Medical University, Chongqing, China
- 3Department of Neurology, Chongqing Hospital of Traditional Chinese Medicine, Chongqing, China
- 4Department of Neurology, Chongqing University Three Gorges Hospital, Chongqing, China
- 5Department of Neurology, The Second Affiliated Hospital of Anhui Medical University, Hefei, Anhui, China
Abstract
The risk factors and causes of intracerebral hemorrhage (ICH) and the degree of functional recovery after ICH are distinct between young and elderly patients. The increasing incidence of ICH in young adults has become a concern; however, research on the molecules and pathways involved ICH in subjects of different ages is lacking. In this study, tandem mass tag (TMT)-based proteomics was utilized to examine the protein expression profiles of perihematomal tissue from young and aged mice 24 h after collagenase-induced ICH. Among the 5,129 quantified proteins, ICH induced 108 and 143 differentially expressed proteins (DEPs) in young and aged mice, respectively; specifically, there were 54 common DEPs, 54 unique DEPs in young mice and 89 unique DEPs in aged mice. In contrast, aging altered the expression of 58 proteins in the brain, resulting in 39 upregulated DEPs and 19 downregulated DEPs. Bioinformatics analysis indicated that ICH activated different proteins in complement pathways, coagulation cascades, the acute phase response, and the iron homeostasis signaling pathway in mice of both age groups. Protein–protein interaction (PPI) analysis and ingenuity pathway analysis (IPA) demonstrated that the unique DEPs in the young and aged mice were related to lipid metabolism and carbohydrate metabolism, respectively. Deeper paired-comparison analysis demonstrated that apolipoprotein M exhibited the most significant change in expression as a result of both aging and ICH. These results help illustrate age-related protein expression changes in the acute phase of ICH.
Impact statement
The increasing incidence of intracerebral hemorrhage (ICH) in young adults has become a concern; however, research on susceptible molecules and pathways specifically targeting ICH in subjects of different ages remains lacking. We aimed to investigate the underlying interaction relationship between aging and ICH by proteomics approaches. In this study, we performed tandem mass tag (TMT)-based proteomics on young and aged mice after constructing collagenase-induced ICH models to screen protein expression in brain perihematomal tissue among groups.
Introduction
Intracerebral hemorrhage (ICH) is a devastating stroke subtype that is associated with low treatable rates and high mortality rates [1]. Approximately 50% of patients die within the first 48 h, and most patients suffer from a severe disability after being discharged from the hospital [2]. Previous studies have revealed that young adult and elderly patients with acute ICH exhibit distinct incidence rates, causes and functional recoveries [3]. Recently, the increasing incidence of ICH in young adults has become a major concern, and permanent disability and death caused by ICH in this demographic bring heavy economic burdens to society and public health organizations [4, 5]. To optimize preventive and protective approaches for young adults with ICH, the pathological changes that occur in young subjects after ICH need to be investigated.
Recently, genome-wide studies of ICH populations have been carried out [6–8]. High-throughput sequencing has provided evidence that age-specific changes occur after ICH events. A proteomics analysis detected differentially expressed proteins (DEPs) in plasma among young, middle-aged, and older healthy individuals and confirmed that insulin-like growth factor 1 is a promising novel approach for ameliorating aging-associated acute brain injury after ICH [9]. The other transcriptomic analysis distinguished the expression pattern of oxylipin enzymes between middle-aged and aged rats 3 days after experimental ICH induction and revealed that among the specific differentially expressed genes (DEGs), Cyp1a1 and Cyp2e1 were the most significant DEGs in the two age groups [10]. In addition, in pathological findings of animals with ICH, more severe brain swelling was observed in aged animals than in young animals, and aged animals exhibited different glial responses from young animals, impaired lesion resolution, and neuronal death [11]. Interestingly, a recent study based on another classic stroke model, middle cerebral artery occlusion mice, found that O-GlcNAcylation was a pro-survival pathway that was impaired in the stroke penumbra in aged mice but was activated in young mice, and this study introduced a therapeutic strategy for treating ischemic stroke in an age-specific manner [12]. However, relatively little is known about the different responses to ICH in aged and young subjects. Therefore, understanding the discrepancies in the mechanisms of internal molecular injury in young and aged patients after ICH is highly desirable.
In ICH, primary injury occurs due to the mechanical compression of the brain parenchyma caused by an expanding intracranial hematoma, resulting in brain edema and neuron damage in the perihematomal area [13]. Furthermore, local ischemia and hypoxia in brain tissue, along with the release of blood components from ruptured blood vessels, initiate secondary brain injury, activating microglia and resident macrophages within the brain and recruiting peripheral leukocytes through the compromised blood‒brain barrier to the perihematomal area, further exacerbating inflammatory injury [14]. Therefore, the perihematomal region is the most commonly studied region in the field of ICH [15–18]. Experimental analysis of perihematomal tissue can provide an in-depth understanding of the mechanisms of secondary injury, the related role of inflammatory mediators, and the processes of neuroprotection and neuronal repair, yielding valuable evidence for the development of strategies for treating brain injury, inhibiting inflammatory responses, and promoting neuroprotection.
This study aimed to explore the differences in protein expression in perihematomal tissue between young and aged mice using proteomics data. The functions and pathways of common and unique DEPs that were identified by comparative proteomics between young and aged mice were investigated, and possible targets and pathways for follow-up studies across different life periods were identified.
Materials and methods
Animals
Specific-pathogen-free 10-week-old and 22-month-old male C57BL/6 mice were purchased from the Laboratory Animal Center of Chongqing Medical University (CQMU). Animals were maintained in a pathogen-free facility and were fed ad libitum in a 12/12-h light-dark environment at 25°C. All experimental protocols were approved by the Ethical Committee of CQMU. All efforts were made to reduce the number of animals and the amount of suffering they endured.
Animal models
The collagenase IV-induced ICH mouse model was established based on a previous study [19]. Anaesthesia was administered to the mice through the inhalation of 4% isoflurane. After remaining under anaesthesia with 1.5% isoflurane, the mice were placed on a stereotaxic apparatus (Rivard, China) in a prone position with a rectal temperature that was maintained at 37.5°C. A 26-gauge microsyringe (Hamilton, the United States) was inserted into the right striatum through an eyehole that was drilled in the skull (the anterior fontanelle was the origin of the coordinate with 0.6 mm anterior and 2.3 mm lateral directions and a 3.7 mm depth). ICH models or sham controls were induced by 0.075 U collagenase (Sigma, the United States) in 0.4 µL of 0.9% physiological saline or an equivalent dose of saline at a rate of 0.5 μL/min. The mice were placed on a warming pad until consciousness was recovered.
Sampling
Mice were randomly divided into a young sham group, a young ICH group, an aged sham group, and an aged ICH group. Twenty-four hours after ICH, the mice were anaesthetized with pentobarbital sodium. Approximately 2 minutes later, after the mice were successfully anaesthetized, they were perfused transcardially with 30 mL sterile phosphate-buffered saline within 6 min. Immediately after perfusion, the brains of the mice were removed, and 1 mm coronal brain sections of perihematomal tissue from the basal ganglia were prepared according to our previous study [20]. The tissues were shock frozen in liquid nitrogen for 30 min and stored at −80°C until use.
Protein extraction and peptide fractionation
Eight mice in each group were used for the proteomic analysis, and 2 or 3 brain samples were pooled as one biological replicate (n = 3 in each group). The samples were lysed in 4% SDS, 10 mM Tris-HCl, and 1 mM DTT (pH = 7.6). Then, the lysates were transferred to a 2 mL centrifuge tube prefilled with an appropriate amount of quartz sand and a 1/4-inch ceramic bead (MP 6540-424), homogenized twice for 120 s (24 × 2, 6.0 m/s) by a FastPrep-24 instrument (MP Biomedicals, the United States) and ultrasonicated 10 times for 100 s (100 W, sonication for 10 s, rest for 5 s). Next, the samples were boiled in water for 15 min and centrifuged at 14,000 g for 30 min, and the supernatant was filtered via 0.22 μm filters. The concentration of the final extracted proteins was quantified using a BCA Protein Assay Kit (Bio-Rad, the United States). Protein digestion and peptide fractionation were performed based on a filter-aided sample preparation (FASP) protocol [21]. In brief, 200 µg of protein sample was incubated with the detergent dithiothreitol to remove residual low-molecular-weight molecules, and then iodoacetamide was added for the alkylation reaction. Peptide concentrations were measured using the ultraviolet spectral density method at 280 nm.
Tandem mass tag (TMT)-based liquid chromatography–mass spectrometry (LC–MS) proteomics analysis
The digested peptides were labelled by the Tandem Mass Tags (TMT) reagent kit (Thermo Scientific, the United States). The label information of 12 samples is shown in Table 1. After TMT labeling, the samples were specifically identified and analyzed using database search software. LC/MS-MS analysis was carried out using Easy nLC Proxeon Biosystems coupled with a Q Exactive mass spectrometer (Thermo Scientific, the United States) for 60/90 min. The digested peptides were loaded onto a nano Viper C18 trap column (100 μm i.d. ×2 cm, Acclaim PepMap100) (Thermo Scientific, the United States) and separated on a capillary C18-reversed-phase analytical column (75 μm i.d. ×10 cm, 3 μm resin, Easy Column) (Thermo Scientific, the United States) with an eluent buffer composed of 0.1% formic acid (FA) and a linear gradient buffer composed of 0.1% FA and 84% acetonitrile at a flow rate of 0.300 μL/min. The 10 most abundant precursor ions were processed during each scan cycle by higher energy collision-induced dissociation (HCD) fragmentation. The automatic gain control (AGC) target value was 3 x e6, and the mass range was 300–1800 m/z. The duration time for the dynamic exclusion was 2/3 min, and the injection time did not exceed 10 ms. The resolutions for the survey scans and HCD spectra were set to 70,000 at m/z 200 and 30,000 at m/z 200, respectively. The underfill rate was 0.1%, the normalized collision energy was adjusted to 30 eV, and the width parameter of isolation was 2 m/z. The mass spectrometer was run in positive mode, and the apparatus was operated in peptide recognition mode.
Protein identification
The raw proteomics data were analyzed by Proteome Discoverer software (v.1.4) using the MASCOT engine (v.2.2) (Matrix Science, Britain). Tandem mass spectra data were matched against the UniProt database1. Trypsin was defined as the cleavage enzyme, and the maximum missing cleavage was 2. The peptide ion mass tolerance was ±20 ppm, and the fragment ion mass tolerance was 0.1 Da. The false discovery rate (FDR) for protein and peptide identification was ≤0.01. Protein quantitative analysis was performed according to the median of the exclusive specific peptides in the MS/MS spectra. The carbamidomethylation of cysteine and the oxidation of methionine were defined as fixed modification and variable modification, respectively. TMT-16plex was used in the quantitation process.
Bioinformatics
The bioinformatics analysis procedure is shown in Figure 1. DEPs between the ICH and sham groups were identified by an FDR <0.05. The subcellular localization of DEPs was searched on CELLO2. The p values and fold change (FC) values of the DEPs were visualized in volcano plots. Hierarchical clustering analyses were conducted by cluster 3.03 and Java Treeview4 and were visualized in heatmaps. The specific and common DEPs between different paired comparisons were identified by Venn plots5. DEP functional analysis was conducted by Gene Ontology (GO) analysis, and pathway analysis was performed by Kyoto Encyclopedia of Genes and Genomes (KEGG)6 and Ingenuity Pathway Analysis (IPA) software (IPA®, Qiagen, the United States)7. Protein–protein interaction (PPI) networks were constructed based on STRING8 and were further analyzed with Cytoscape software by the MCODE and CytoNCA plug-ins9.
Results
Identification of DEPs in young and aged mice after ICH
The number of obtained spectra, peptides, and proteins are presented in Table 2. Specifically, the expression of a total of 5,129 proteins in the brains of young and aged mice was quantified, and the accession numbers, expression levels, sequence coverages and number of peptides identified are listed in Supplementary Tables S1, S2. Next, we examined the differences in the protein expression profiles of perihematomal tissues after ICH between young and aged mice by performing DEP analysis at 24 h after ICH and comparing the spectra with those of age-matched sham controls. When the DEPs were screened by FC > 1 and <1 and FDR <0.05, the FCs in DEPs between the ICH mice and sham controls in both age groups displayed significant discrepancies, as seen in the hierarchical clustering heatmaps, which grouped the samples from the ICH group and sham controls into two different clusters and enabled us to perform the following analysis (Supplementary Figures S1A, S1B). The full names of the DEPs in the heatmaps are presented in Supplementary Table S3. As depicted in the volcano plot (Figure 2A), ICH upregulated 95 and downregulated 13 proteins in young mice. In contrast, there were 119 upregulated proteins and 24 downregulated proteins in aged mice after ICH (Figure 2B). As shown in Figure 2C, common and unique DEPs were identified by a Venn diagram and included 54 unique DEPs in young mice (41 upregulated and 13 downregulated in the ICH group relative to controls), 89 unique DEPs in aged mice (65 upregulated and 24 downregulated in the ICH group relative to controls), and 54 common DEPs (all upregulated in the ICH group relative to controls) in the two age groups. To reduce the number of DEPs, FC > 2, FDR <0.05 was set as a stricter threshold to identify the most significant DEPs. The subcellular localization and biological function of the DEPs are described in detail in Supplementary Table S4.
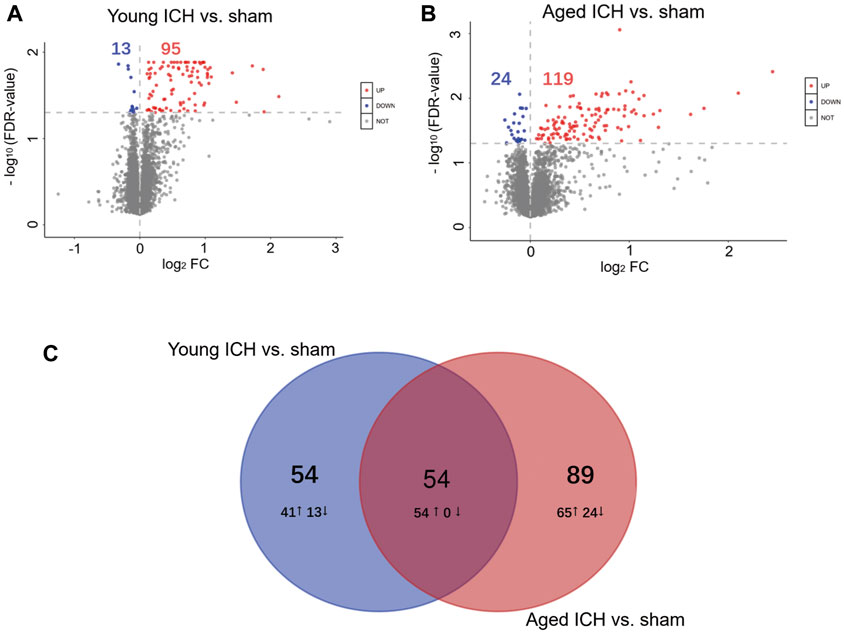
Figure 2. Identification of DEPs and PPI analysis. Volcano plots of DEPs between the ICH and sham groups in (A) young and (B) aged mice. (C) Venn diagram analysis of the ICH-induced DEPs between different age-matched comparisons.
Identification of specific and common DEP-related functions and pathways
To gain precise and thorough insight into the effect of aging and ICH, we explored the PPIs and performed module cluster analysis by GO enrichment and KEGG pathway analysis for specific and common DEPs in aged and young mice. To prevent the possibility of omitting meaningful functions and pathways due to false-negative results, the criterion for the identification of DEPs was once again set as FC > 1 and <1 and FDR <0.05.
Next, PPI analysis was conducted. As shown in Figure 3A, most of the common DEPs were clustered in one module, and their functions were associated with complement and coagulation cascades (Supplementary Table S5). The two modules in the PPI network constructed from unique DEPs in young mice were associated with negative regulation of the remodeling of very-low-density lipoprotein particles and ribosomal subunits (Figure 3B; Supplementary Table S5). In contrast, the DEPs constructed from unique DEPs in aged mice were divided into 3 modules, which were related to complement and coagulation cascades, glycolytic processes, and the biosynthetic processes of porphyrin-containing compounds (Figure 3C; Supplementary Table S5).
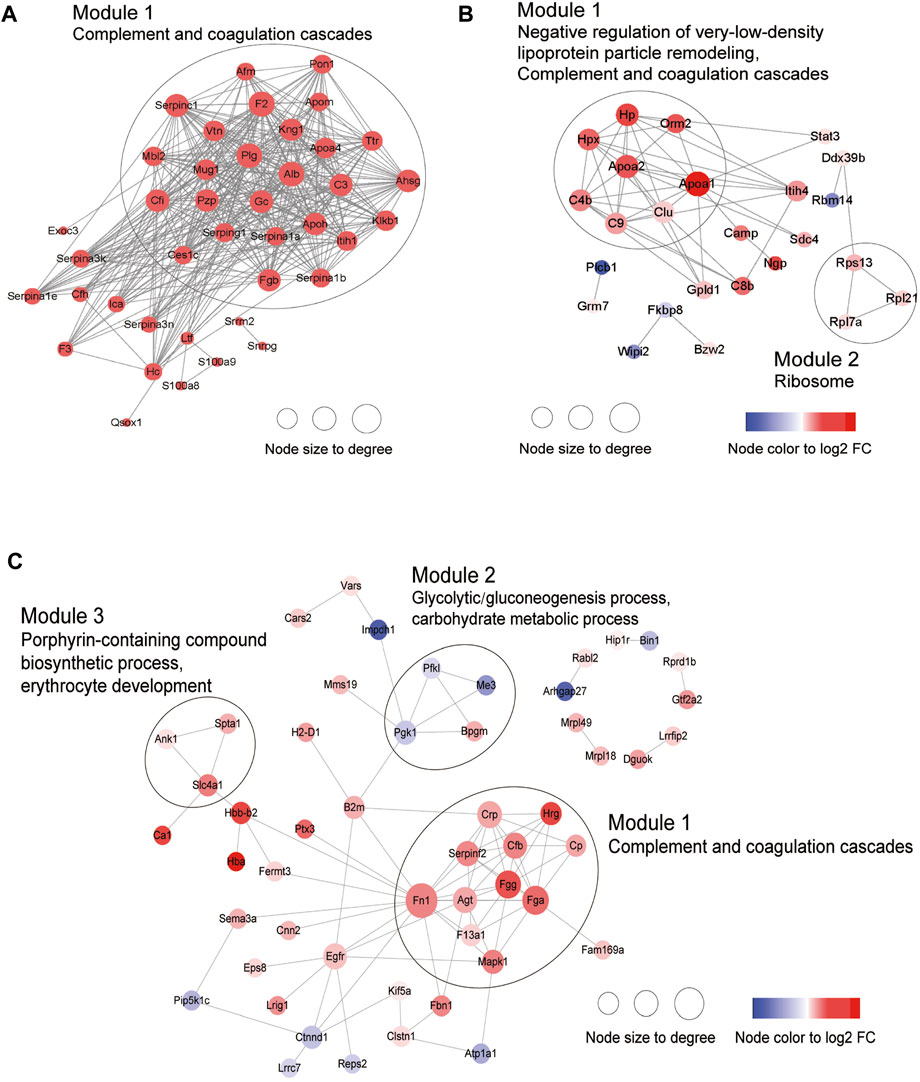
Figure 3. Construction of PPI network. (A) Common DEPs and (B) Specific DEPs in young and (C) aged mice after ICH.
Identification of specific and common up- or downregulated DEP-related functions and pathways
Then, the ICH-induced DEPs in the two age groups were further divided into upregulated and downregulated DEPs for IPA. Consistent with the functional analysis of module clusters from PPI networks, biofunctional analysis conducted via IPA software demonstrated that lipid metabolism and carbohydrate embolism were significantly activated after ICH in young and aged mice, respectively (Supplementary Figures S2A, S2B). In the results for the IPA pathway enrichment of upregulated DEPs, the signaling pathways for iron homeostasis and pancreatic adenocarcinoma were the common activated pathways (Figures 4A–C; Supplementary Table S6). However, the proteins involved in the iron homeostasis signaling pathway were different. The proteins that participated in this pathway included STAT3, HPX, and HP from young mice and CP, EGFR, Hbb-b1, Hbb-b2, MAPK1, and MMS19 from aged mice (Figures 5, 6). In addition, regarding neurodegenerative diseases (NDDs), several functions, including Huntington’s disease signaling (EGFR, MAPK1, PSMB3), neuroprotection by THOP1 in Alzheimer’s disease (AGT, MAPK1) and Parkinson’s signaling (MAPK1), were activated only in aged mice (Supplementary Table S6). The shared pathways among the three clusters of DEPs included the complement and coagulation system and acute phase response, and this finding was consistent with the PPI analysis (Figures 4A–C; Supplementary Table S6). For the downregulated DEPs, D-IP3 biosynthesis and autophagy were the common functional pathways that were activated (Supplementary Table S6).
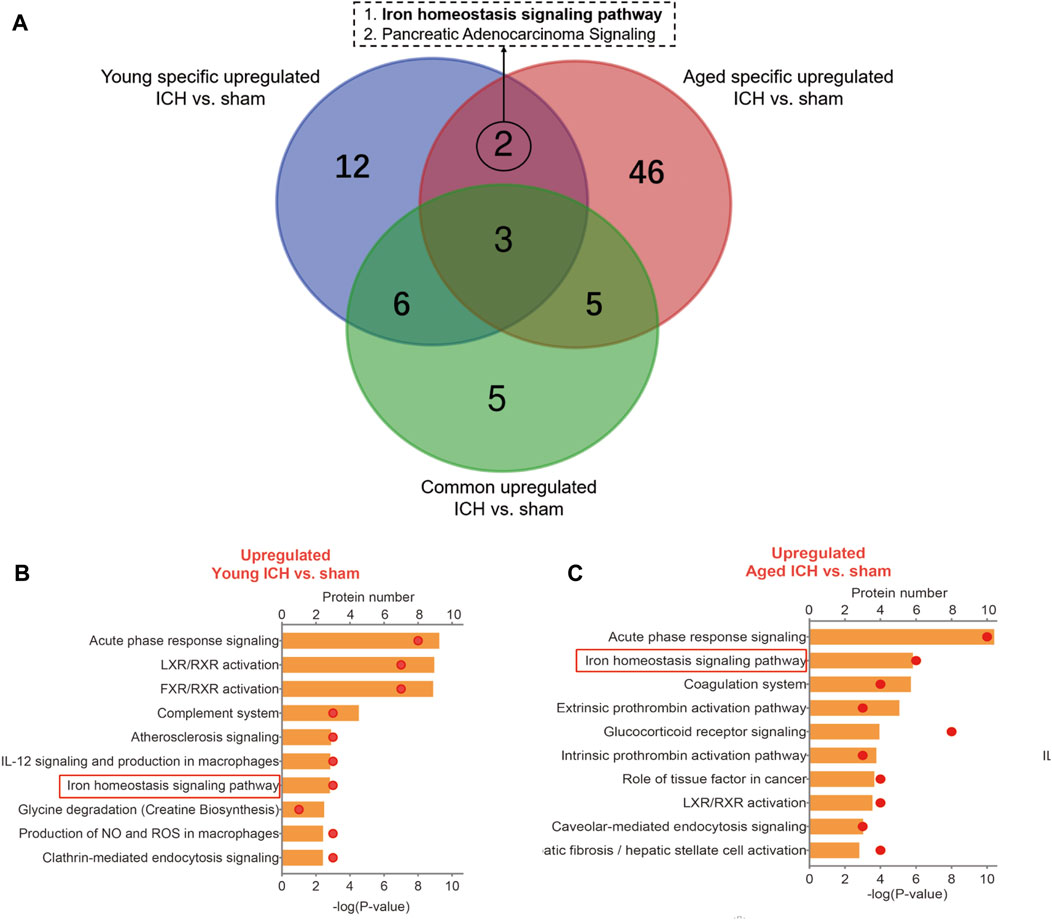
Figure 4. IPA analysis of specific upregulated DEPs. (A) The number of IPA pathways between specific and common upregulated DEPs in aged or young mice after ICH. (B) The top 10 IPA pathways in young and (C) aged mice after ICH compared with the corresponding sham controls.
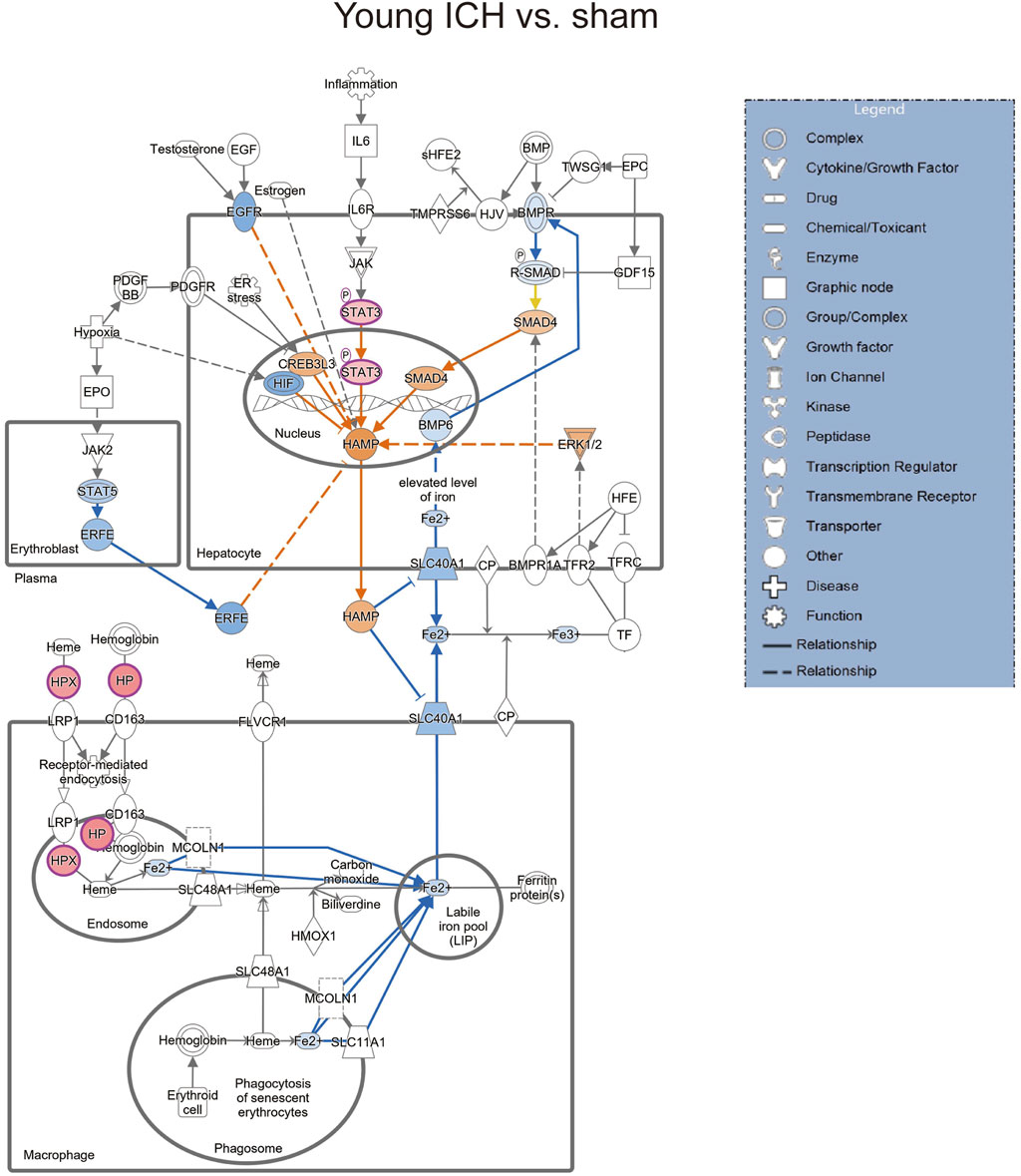
Figure 5. The iron homeostasis signaling pathway in young mice after ICH compared with the corresponding sham controls (by IPA software).
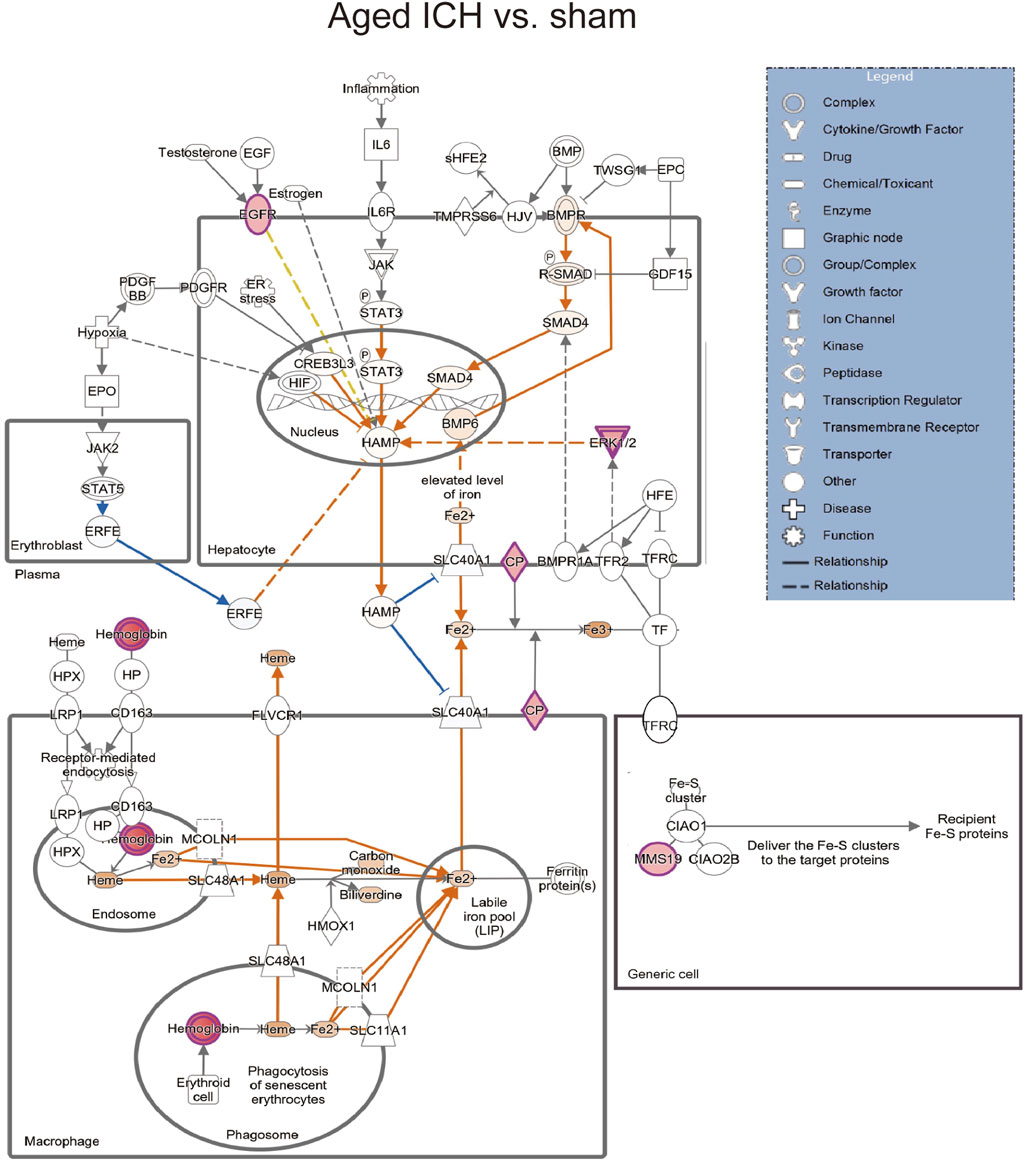
Figure 6. The iron homeostasis signaling pathway in aged mice after ICH compared with the corresponding sham controls (by IPA software).
We next explored the proinflammatory factors that correlated with proteins of the iron homeostasis signaling pathway in both age groups. The networks obtained with the IPA software and constructed based on the direct and indirect relationships among the unique upregulated DEPs in the two age groups demonstrated that IL1 was the upstream molecule of HP in the young mice after ICH (Figure 7A) and that the NF-κB complex interacted with Hbb-b1 in aged mice after ICH (Figure 7B).
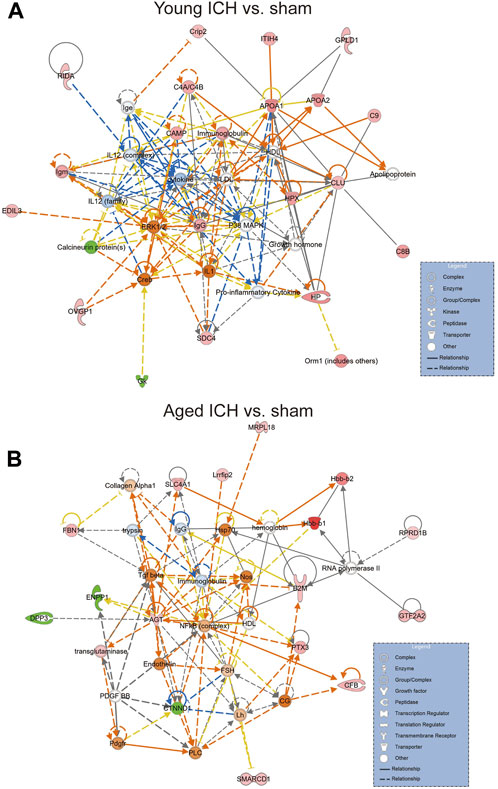
Figure 7. The internet interaction of DEPs in young and aged mice after ICH (by IPA software). (A) Young ICH vs. Young sham. (B) Aged ICH vs. Aged sham.
Specific markers between aged and young mice after ICH
Considering that the previous comparison was limited to ICH vs. sham, some important markers may have been missed. Thus, we explored different pairwise-comparison groups to explore the proteins affected by age alone and those affected by both age and ICH. As shown in Figures 8A, B, the expression of Bsg, Ptgfrn, Dpysl3, Rras2, Prdx1, Gnpda2, Gja1, Folh1, Serinc5, C1qtnf5, Ccdc8, Vcan, Nmral1, Fth1, Hlta1, Mog, Hapln2, and Cldn11 was affected by age only (18 in total), and that of ApoM, Psmb2, Ttr, Kng1, and Serpina3k was affected by both age and ICH (5 in total).
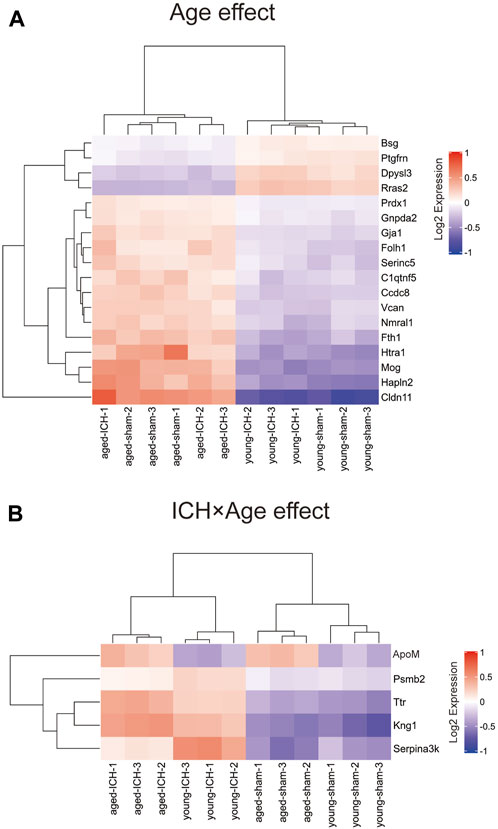
Figure 8. DEPs affected by age or ICH. (A) DEPs that were affected by age only. (B) DEPs that were affected by age and ICH.
DEP analysis between the aged and young sham groups
To exclude the inherent differences between aged and young mice, we further compared the DEPs between the two sham groups. A total of 58 DEPs were identified between the aged and young sham groups of mice (Figure 9A; Supplementary Figure S3). The full names of the DEPs in the heatmap are presented in Supplementary Table S3. Aging downregulated 19 proteins in the basal ganglia, including the SH3 domain binding proteins Dpysl3, Shank2, and Mical1, the dendrite development-related proteins Srcin1 and Cyth2 and the neuron differentiation-related proteins Dpysl3, Shank2, Slc23a2 and Brinp2 (Figure 9B). Moreover, 39 proteins were upregulated in the brains of aged mice compared to those of young controls, and the functions of these proteins were identified by KEGG and GO enrichment analysis11 (last accessed on 10 November 2023, an online platform for data analysis and visualization) (Figure 9C; Supplementary Table S7) [22–24]. According to GO analysis, the DEPs were significantly enriched in 392 biological process (BP) terms (p < 0.05), with cellular modified amino acid metabolicprocess, regulation of organelle transport along microtubule, and astrocyte differentiation being the top BP terms. Furthermore, these DEPs were significantly enriched in 61 GO cellular component (CC) terms. Notably, the top CC terms included myelin sheath, lateral plasma membrane, and basal part of cell. Moreover, 71 molecular function (MF) terms were significantly overrepresented, including toxic substance binding, carboxylic acid binding, and organic acid binding. Additionally, KEGG pathway analysis revealed 11 significantly enriched pathways, including alanine, aspartate and glutamate metabolism, retrograde endocannabinoid signaling, and hepatitis C.
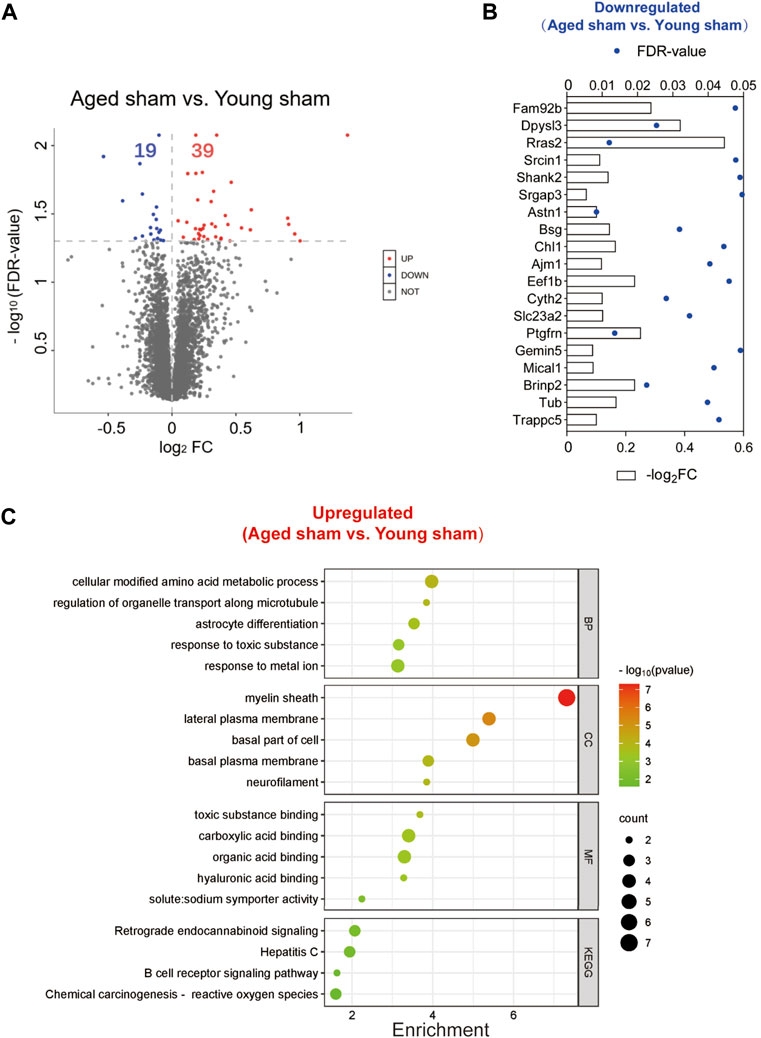
Figure 9. Identification of DEPs between the aged and young sham groups and functional enrichment analysis. (A) Volcano plot of the 58 DEPs in the aged sham group versus the young sham group. (B) Expression of the 19 downregulated DEPs between the two sham groups. (C) Top GO terms and KEGG pathways enriched in the 39 upregulated DEPs between the two sham groups.
Then, we performed PPI and module analysis of all DEPs (Figure 10; Supplementary Table S5). The proteins in module 1 were primarily associated with GO-BP terms such as axon ensheathment, cell adhesion, and glial cell differentiation. On the other hand, the proteins in module 2 were mainly involved in mitochondrial ATP synthesis-coupled proton transport.
Discussion
In the current study, we conducted LC–MS/MS analysis to examine changes in the protein profile of perihematomal tissue in response to ICH in the brains of aged and young mice. The functional analysis of age-specific DEPs and common DEPs in different ages implied that different age-related response patterns occurred at the acute phase of brain injury after ICH. Decoding aberrant protein expression patterns between young and aged groups of ICH mice can provide age-related internal vulnerable molecular mechanisms.
Specific and common DEPs in both age groups were associated with complement and coagulation cascades and the acute phase response, and these pathways are known as the vital BP that occurs with brain injury after ICH. Surprisingly, we found that the clustered DEPs that were specific to aged and young mice were enriched in glycolysis/gluconeogenesis processes and ribosomal subunits, respectively. Enhanced glycolysis/gluconeogenesis could promote the proinflammatory function of macrophages [25, 26]. In aged mice after ICH, decreased Pfkl (ATP-dependent 6-phosphofructokinase, liver type) and Pgk1 (phosphoglycerate kinase 1) proteins and increased Bpgm (bisphosphoglycerate mutase) protein were clustered together, and their functions involved glycolysis/gluconeogenesis and carbohydrate metabolic processes. Pfkl is a subtype of Pfk, and in a previous transcriptomics study, the elevated level of Pfk gene expression in CD14+ monocytes/macrophages from hematoma tissue was reported to be significantly associated with good outcomes for ICH patients at the subacute stage [27]. Clinical and preclinical data have shown that increased Pgk1 activity and glycolysis ameliorate the neurodegeneration and progression of Parkinson’s disease [28]. Bpgm plays an essential role in reversing aging-related cognitive impairment and auditory dysfunction [29]. Based on these findings, the significant change in glycolysis protein levels in the acute stage of ICH may be a specific predictor of poor outcomes. In addition, the other module from the aged-specific DEP PPI network included Spta1 (spectrin alpha chain, erythrocytic 1), Slc4a1 (band 3 anion transport protein), and Ank1 (ankyrin-1). In clinical research, the Slc4a1 and Ank1 genes from the peripheral blood of ICH patients were verified as hub genes by RNA sequence analysis, and this result implied that these genes have the potential to be used as key intervention targets in translational research [30].
In contrast, lipid metabolism was significantly affected in the young mice. Oxylipin profiling at 12 h, 24 h, and 72 h after ICH in adult mouse brains was explored in a recent study, and the number of changed oxylipins was the highest on the first day of ICH [10]. In the present study, the expression of the apolipoprotein A-I (Apoa1) protein differed between young and aged ICH model mice, being significantly elevated exclusively in young mice. This discrepancy may be associated with the weaker response to ICH in aged mice. Apoa1, as a component of high-density lipoprotein, may exert a protective effect by reducing cholesterol deposition and inhibiting inflammatory responses. Previous studies have also identified it as a protective factor in ICH, supporting this hypothesis [31, 32]. Consequently, in aged mice and even patients with ICH, greater attention should be paid to changes in the level of this indicator and related treatments. However, the interaction between lipid metabolism and ICH is still worthy of further exploration. In addition, the levels of Rps13 (40S ribosomal protein S13), Rpl21 (60S ribosomal protein L21), and Rpl7a (60S ribosomal protein L7a) genes were elevated, and these genes interacted with each other in the PPI network that was constructed by DEPs from young mice after ICH. The genes are all components of ribosomal subunits. Ribosomal damage could cause the TP53 pathway to be disrupted and increase ROS [33], which have a close link with secondary brain injury after ICH [34]. Significantly, Rpl7a was identified as a hub gene in a murine model of traumatic brain injury [35], and increased Rps13 gene levels in microglia and epithelial cells were found to be aging-related in a single-cell sequence study on murine brains [36]. In our study, the elevated ribosomal-associated proteins functioned as transcriptional proteins, and they may play a role in brain injury in young mice after ICH.
Secondary brain injury includes erythrocyte lysis with the production of neurotoxic iron ions, immune-inflammatory reactions, and coagulation cascades, which result in nerve cell death and brain tissue necrosis [37]. Previous studies have shown that the distribution of iron in the healthy human brain is region-specific and that its concentration increases with age [38, 39]. The putamen nucleus, caudate nucleus, and globus pallidus in the basal ganglia are the brain regions with the highest concentration of iron [40]. The increase in the number of glial cells and the permeability of the blood–brain barrier with age may explain the accumulation of iron in the basal ganglia. In the present proteomics analysis, we found that the signaling pathway of iron homeostasis was activated after ICH in both aged and young mice. Interestingly, the number and type of proteins that were activated in the pathway were significantly higher in the aged mice after ICH than in the young mice. Following ICH, the proteins involved in iron metabolism in young mice include HP (haptoglobin), HPX (hemopexin), and STAT3 (signal transducer and activator of transcription 3). On the other hand, in aged mice, the proteins related to iron metabolism were CP (ceruloplasmin), EGFR (epidermal growth factor receptor), Hbb-b1 (hemoglobin subunit beta-1), Hbb-b2 (hemoglobin subunit beta-2), MAPK1 (mitogen-activated protein kinase 1), and MMS19 (MMS19 nucleotide excision repair protein). HP and HPX and CP are plasma proteins synthesized by the liver that can bind to hemoglobin, heme and copper, respectively. Previous studies have suggested that increasing HPX and CP levels or reducing HP levels could be potential strategies for the treatment of experimental ICH [41–43]. Specifically, the therapeutic effect of HP gene knockout on ICH was age dependent, with Hp depletion resulting in a better neurological outcome in young ICH model mice but being ineffective in aged ICH model mice. Similarly, another experiment on age-related memory decline showed that specific knockout of CP in astrocytes had a beneficial effect on aged mice but had detrimental effects on young mice [44]. These results emphasize the importance of considering age-related factors when developing strategies for protecting the brain and indicate that the underlying mechanisms of ICH and response to treatment may differ between young and aged individuals. When iron metabolism becomes dysfunctional, excess iron and hydroxyl radicals are produced, resulting in OS damage and neuronal injury or death [45]. Previous studies based on an ICH model with aged murine and aged rats indicated that mediating iron metabolism and reducing iron accumulation could alleviate neuronal death and neurological deficits [46, 47]. The present results suggest that after ICH in aged individuals, iron metabolism is a crucial process in the brain injury mechanism. In addition, several DEPs in the aged mice were enriched in NDD-related pathways, which suggests a possible interactive correlation between ICH and NDD in pathological mechanisms.
To select meaningful molecular markers for validation, we performed additional pairwise comparisons. Among the proteins altered by age alone, Cldn11 (claudin-11) and Mog(myelinoligodendrocyte glycoprotein are myelin-associated proteins. ApoM (apolipoprotein M), Psmb2 (proteasome subunit beta type-2), Ttr (transthyretin), Kng1 (kininogen-1) and Serpina3k (serine protease inhibitor A3K) were altered by both age and ICH. Indeed, disruption of myelin-related molecules with age has been demonstrated in previous experiments [48]. ApoM binding to sphingosine-1-phosphate has been reported to have inhibitory effects on lymphopoiesis and neuroinflammation [49]. Treatments targeting Ttr and Serpina3k exerted significant protective effects against experimental traumatic brain injury [50, 51]. Kng1 is involved in regulating vasodilation and inflammatory responses [52], while Psmb1 functions in the degradation and processing of intracellular proteins [53]. The dysregulation of these two proteins may indicate a pronounced change in the inflammatory response due to age and ICH. Considering these findings together, the higher Serpina3k protein level in younger mice may explain the better long-term prognosis of younger subjects after ICH, and the higher ApoM and Ttr levels in elderly subjects with ICH may be due to a compensatory mechanism for protecting against acute brain injury after ICH.
The inclusion of the two sham groups in this study has further improved our understanding of the impact of aging on the brain. Aging is a complex BP involving changes at multiple levels, including disruptions of cellular metabolism [54]. According to our results, the dysregulation of modified amino acid metabolism within cells is associated with aging. During the aging process, the metabolic activity and regulatory capacity of cells gradually decline, which can lead to abnormalities in the synthesis, degradation, and conversion of modified amino acids [55]. For instance, research has found that in aging cells, there is an abnormal increase or decrease in processes such as methylation and acetylation, which can result in changes in protein function and aberrant cellular metabolism [56]. Additionally, alterations in the expression of myelin proteins and changes in the regulation of proteins involved in myelination during aging were observed. Myelin plays a crucial role in facilitating efficient nerve signal transmission, and any disturbance of or deterioration in its structure or composition may impact neural communication [57]. A previous study demonstrated that significant alterations occurred in sphingolipid expression patterns associated with myelin remodeling during the aging process [58]. With advancing age, mitochondrial ATP synthesis coupled proton transport was also affected. This could be attributed to changes in the internal structure and function of mitochondria, including mitochondrial DNA damage, membrane instability, and increased oxidative stress [59–61]. These factors may contribute to a decrease in the mitochondrial membrane potential, consequently affecting the efficiency of proton pump function and ATP synthesis.
Taken together, the results of our current study provide information on specific DEPs and their functions in mice of different ages after ICH, contribute to the exploration of the age-specific approach for ICH and provide foundations for future research on the subject.
Author contributions
XL, QL, and PX designed the experiment. XL, ZX, PL, WY, YS, FL, XX, QW, PW, and RD performed the experiments. XL wrote the draft manuscript. QL, PX, and WY revised the manuscript. All authors collected and analyzed the data and approved the submitted version.
Data availability statement
The original contributions presented in the study are included in the article/Supplementary Material, further inquiries can be directed to the corresponding authors. The MS proteomics data have been deposited in the ProteomeXchange Consortium http://proteomecentral.proteomexchange.org via the iProX partner repository with the dataset identifier PXD033791 [62].
Ethics statement
The animal study was approved by the Ethical Committee of Chongqing Medical University (CQMU). The study was conducted in accordance with the local legislation and institutional requirements.
Funding
The author(s) declare financial support was received for the research, authorship, and/or publication of this article. This study was supported by the National Key R&D Program of China (Grant No. 2017YFA0505700), the National Natural Science Foundation of China (No. 82071337), the National Key R&D Program of China (Nos. 2018YFC1312200 and 2018YFC1312203), the Chongqing High-end Young Investigator Project (No. 2019GDRC005), the Science and Technology Innovation Project of “Chengdu Chongqing Economic Circle” (No. KJCXZD2020019), the Excellent Youth Foundation of Chongqing Scientific Committee (cstc2021jcyj-jqX0029), the Non-profit Central Research Institute Fund of Chinese Academy of Medical Sciences (Grant No. 2019PT320002), and the National Administration of Traditional Chinese Medicine: 2019 Project of Building Evidence-Based Practice Capacity for TCM (No. 2019XZZX-NB014).
Acknowledgments
We thank Shanghai NewCore Biotechnology Co., Ltd. (https://www.bioinformatics.com.cn, last accessed on 10 November 2023) for providing data analysis and visualization support.
Conflict of interest
The authors declare that the research was conducted in the absence of any commercial or financial relationships that could be construed as a potential conflict of interest.
Supplementary material
The Supplementary Material for this article can be found online at: https://www.ebm-journal.org/articles/10.3389/ebm.2024.10117/full#supplementary-material
Footnotes
2http://cello.life.nctu.edu.tw/
3http://bonsai.hgc.jp/∼mdehoon/software/cluster/software.htm
4http://jtreeview.sourceforge.net
5http://bioinformatics.psb.ugent.be/webtools/Venn/
6https://david.ncifcrf.gov/summary.jsp
7https://www.qiagenbioinformatics.com
9https://apps.cytoscape.org/apps/
11https://www.bioinformatics.com.cn
References
1. Cordonnier, C, Demchuk, A, Ziai, W, and Anderson, CS. Intracerebral haemorrhage: current approaches to acute management. The Lancet (2018) 392(10154):1257–68. doi:10.1016/s0140-6736(18)31878-6
2. Zia, E, Engstrom, G, Svensson, PJ, Norrving, B, and Pessah-Rasmussen, H. Three-year survival and stroke recurrence rates in patients with primary intracerebral hemorrhage. Stroke (2009) 40(11):3567–73. doi:10.1161/strokeaha.109.556324
3. Tatlisumak, T, Cucchiara, B, Kuroda, S, Kasner, SE, and Putaala, J. Nontraumatic intracerebral haemorrhage in young adults. Nat Rev Neurol (2018) 14(4):237–50. doi:10.1038/nrneurol.2018.17
4. Bako, AT, Pan, A, Potter, T, Tannous, J, Johnson, C, Baig, E, et al. Contemporary trends in the nationwide incidence of primary intracerebral hemorrhage. Stroke (2022) 53(3):e70–e74. doi:10.1161/strokeaha.121.037332
5. Koivunen, RJ, Satopaa, J, Meretoja, A, Strbian, D, Haapaniemi, E, Niemela, M, et al. Incidence, risk factors, etiology, severity and short-term outcome of non-traumatic intracerebral hemorrhage in young adults. Eur J Neurol (2015) 22(1):123–32. doi:10.1111/ene.12543
6. Hauer, AJ, Kleinloog, R, Giuliani, F, Rinkel, G, de Kort, GA, Berkelbach van der Sprenkel, JW, et al. RNA-sequencing highlights inflammation and impaired integrity of the vascular wall in brain arteriovenous malformations. Stroke (2020) 51(1):268–74. doi:10.1161/strokeaha.119.025657
7. Li, Z, Li, Y, Han, J, Zhu, Z, Li, M, Liu, Q, et al. Formyl peptide receptor 1 signaling potentiates inflammatory brain injury. Sci Transl Med (2021) 13(605):eabe9890. doi:10.1126/scitranslmed.abe9890
8. Winkler, EA, Kim, CN, Ross, JM, Garcia, JH, Gil, E, Oh, I, et al. A single-cell atlas of the normal and malformed human brain vasculature. Science (2022) 375(6584):eabi7377. doi:10.1126/science.abi7377
9. Yuan, JJ, Zhang, Q, Gong, CX, Wang, FX, Huang, JC, Yang, GQ, et al. Young plasma ameliorates aging-related acute brain injury after intracerebral hemorrhage. Biosci Rep (2019) 39(5):BSR20190537. doi:10.1042/bsr20190537
10. Yuan, JJ, Chen, Q, Xiong, XY, Zhang, Q, Xie, Q, Huang, JC, et al. Quantitative profiling of oxylipins in acute experimental intracerebral hemorrhage. Front Neurosci (2020) 14:777. doi:10.3389/fnins.2020.00777
11. Wasserman, JK, Yang, H, and Schlichter, LC. Glial responses, neuron death and lesion resolution after intracerebral hemorrhage in young vs aged rats. Eur J Neurosci (2008) 28(7):1316–28. doi:10.1111/j.1460-9568.2008.06442.x
12. Jiang, M, Yu, S, Yu, Z, Sheng, H, Li, Y, Liu, S, et al. XBP1 (X-Box-Binding protein-1)-dependent O-GlcNAcylation is neuroprotective in ischemic stroke in young mice and its impairment in aged mice is rescued by thiamet-G. Stroke (2017) 48(6):1646–54. doi:10.1161/strokeaha.117.016579
13. Zille, M, Farr, TD, Keep, RF, Romer, C, Xi, G, and Boltze, J. Novel targets, treatments, and advanced models for intracerebral haemorrhage. EBioMedicine (2022) 76:103880. doi:10.1016/j.ebiom.2022.103880
14. Magid-Bernstein, J, Girard, R, Polster, S, Srinath, A, Romanos, S, Awad, IA, et al. Cerebral hemorrhage: pathophysiology, treatment, and future directions. Circ Res (2022) 130(8):1204–29. doi:10.1161/circresaha.121.319949
15. Puy, L, Perbet, R, Figeac, M, Duchene, B, Deramecourt, V, Cordonnier, C, et al. Brain peri-hematomal area, a strategic interface for blood clearance: a human neuropathological and transcriptomic study. Stroke (2022) 53(6):2026–35. doi:10.1161/strokeaha.121.037751
16. Li, H, Ghorbani, S, Zhang, R, Ebacher, V, Stephenson, EL, Keough, MB, et al. Prominent elevation of extracellular matrix molecules in intracerebral hemorrhage. Front Mol Neurosci (2023) 16:1251432. doi:10.3389/fnmol.2023.1251432
17. Tang, L, Wang, L, Jin, F, Hao, Y, Zhao, T, Zheng, W, et al. Inflammatory regulation by restraining M2 microglial polarization: neurodestructive effects of Kallikrein-related peptidase 8 activation in intracerebral hemorrhage. Int Immunopharmacology (2023) 124(Pt A):110855. doi:10.1016/j.intimp.2023.110855
18. Zhuang, J, Peng, Y, Gu, C, Chen, H, Lin, Z, Zhou, H, et al. Wogonin accelerates hematoma clearance and improves neurological outcome via the PPAR-gamma pathway after intracerebral hemorrhage. Transl Stroke Res (2021) 12(4):660–75. doi:10.1007/s12975-020-00842-9
19. Bobinger, T, Manaenko, A, Burkardt, P, Beuscher, V, Sprügel, MI, Roeder, SS, et al. Siponimod (BAF-312) attenuates perihemorrhagic edema and improves survival in experimental intracerebral hemorrhage. Stroke (2019) 50(11):3246–54. doi:10.1161/strokeaha.119.027134
20. Yang, WS, Shen, YQ, Yang, X, Li, XH, Xu, SH, Zhao, LB, et al. MicroRNA transcriptomics analysis identifies dysregulated hedgehog signaling pathway in a mouse model of acute intracerebral hemorrhage exposed to hyperglycemia. J Stroke Cerebrovasc Dis (2022) 31(3):106281. doi:10.1016/j.jstrokecerebrovasdis.2021.106281
21. Wiśniewski, JR, Zougman, A, Nagaraj, N, and Mann, M. Universal sample preparation method for proteome analysis. Nat Methods (2009) 6(5):359–62. doi:10.1038/nmeth.1322
22. Tang, D, Chen, M, Huang, X, Zhang, G, Zeng, L, Zhang, G, et al. SRplot: a free online platform for data visualization and graphing. PLoS One (2023) 18(11):e0294236. doi:10.1371/journal.pone.0294236
23. Yu, G, Wang, LG, Han, Y, and He, QY. clusterProfiler: an R package for comparing biological themes among gene clusters. OMICS: A J Integr Biol (2012) 16(5):284–7. doi:10.1089/omi.2011.0118
24. Luo, W, and Brouwer, C. Pathview: an R/Bioconductor package for pathway-based data integration and visualization. Bioinformatics (2013) 29(14):1830–1. doi:10.1093/bioinformatics/btt285
25. Jung, J, Zeng, H, and Horng, T. Metabolism as a guiding force for immunity. Nat Cel Biol (2019) 21(1):85–93. doi:10.1038/s41556-018-0217-x
26. Van den Bossche, J, O’Neill, LA, and Menon, D. Macrophage immunometabolism: where are we (going)? Trends Immunol (2017) 38(6):395–406. doi:10.1016/j.it.2017.03.001
27. Askenase, MH, Goods, BA, Beatty, HE, Steinschneider, AF, Velazquez, SE, Osherov, A, et al. Longitudinal transcriptomics define the stages of myeloid activation in the living human brain after intracerebral hemorrhage. Sci Immunol (2021) 6(56):eabd6279. doi:10.1126/sciimmunol.abd6279
28. Cai, R, Zhang, Y, Simmering, JE, Schultz, JL, Li, Y, FernandezCarasa, I, et al. Enhancing glycolysis attenuates Parkinson's disease progression in models and clinical databases. J Clin Invest (2019) 129(10):4539–49. doi:10.1172/jci129987
29. Qiang, Q, Manalo, JM, Sun, H, Zhang, Y, Song, A, Wen, AQ, et al. Erythrocyte adenosine A2B receptor prevents cognitive and auditory dysfunction by promoting hypoxic and metabolic reprogramming. PLoS Biol (2021) 19(6):e3001239. doi:10.1371/journal.pbio.3001239
30. Durocher, M, Ander, BP, Jickling, G, Hamade, F, Hull, H, Knepp, B, et al. Inflammatory, regulatory, and autophagy co-expression modules and hub genes underlie the peripheral immune response to human intracerebral hemorrhage. J neuroinflammation (2019) 16(1):56. doi:10.1186/s12974-019-1433-4
31. O'Donnell, MJ, McQueen, M, Sniderman, A, Pare, G, Wang, X, Hankey, GJ, et al. Association of lipids, lipoproteins, and apolipoproteins with stroke subtypes in an international Case control study (INTERSTROKE). J Stroke (2022) 24(2):224–35. doi:10.5853/jos.2021.02152
32. Alsamani, R, Limin, Z, Jianwei, W, Dan, W, Yuehong, S, Ziwei, L, et al. Predictive value of the apolipoprotein B/A1 ratio in intracerebral hemorrhage outcomes. J Clin Lab Anal (2022) 36(7):e24562. doi:10.1002/jcla.24562
33. Sulima, S, Kampen, K, and De Keersmaecker, K. Cancer biogenesis in ribosomopathies. Cells (2019) 8(3):229. doi:10.3390/cells8030229
34. Stockwell, BR, Friedmann Angeli, JP, Bayir, H, Bush, AI, Conrad, M, Dixon, SJ, et al. Ferroptosis: a regulated cell death nexus linking metabolism, redox biology, and disease. Cell (2017) 171(2):273–85. doi:10.1016/j.cell.2017.09.021
35. Ma, Y, Liu, Y, Ruan, X, Liu, X, Zheng, J, Teng, H, et al. Gene expression signature of traumatic brain injury. Front Genet (2021) 12:646436. doi:10.3389/fgene.2021.646436
36. Ximerakis, M, Lipnick, SL, Innes, BT, Simmons, SK, Adiconis, X, Dionne, D, et al. Single-cell transcriptomic profiling of the aging mouse brain. Nat Neurosci (2019) 22(10):1696–708. doi:10.1038/s41593-019-0491-3
37. Shi, K, Tian, DC, Li, ZG, Ducruet, AF, Lawton, MT, and Shi, FD. Global brain inflammation in stroke. Lancet Neurol (2019) 18(11):1058–66. doi:10.1016/s1474-4422(19)30078-x
38. Ramos, P, Santos, A, Pinto, NR, Mendes, R, Magalhães, T, and Almeida, A. Iron levels in the human brain: a post-mortem study of anatomical region differences and age-related changes. J Trace Elem Med Biol (2014) 28(1):13–7. doi:10.1016/j.jtemb.2013.08.001
39. Aquino, D, Bizzi, A, Grisoli, M, Garavaglia, B, Bruzzone, MG, Nardocci, N, et al. Age-related iron deposition in the basal ganglia: quantitative analysis in healthy subjects. Radiology (2009) 252(1):165–72. doi:10.1148/radiol.2522081399
40. Farrall, AJ, and Wardlaw, JM. Blood-brain barrier: ageing and microvascular disease--systematic review and meta-analysis. Neurobiol Aging (2009) 30(3):337–52. doi:10.1016/j.neurobiolaging.2007.07.015
41. Leclerc, JL, Santiago-Moreno, J, Dang, A, Lampert, AS, Cruz, PE, Rosario, AM, et al. Increased brain hemopexin levels improve outcomes after intracerebral hemorrhage. J Cereb Blood Flow Metab (2018) 38(6):1032–46. doi:10.1177/0271678x16679170
42. Leclerc, JL, Li, C, Jean, S, Lampert, AS, Amador, CL, Diller, MA, et al. Temporal and age-dependent effects of haptoglobin deletion on intracerebral hemorrhage-induced brain damage and neurobehavioral outcomes. Exp Neurol (2019) 317:22–33. doi:10.1016/j.expneurol.2019.01.011
43. Liu, H, Hua, Y, Keep, RF, and Xi, G. Brain ceruloplasmin expression after experimental intracerebral hemorrhage and protection against iron-induced brain injury. Transl Stroke Res (2019) 10(1):112–9. doi:10.1007/s12975-018-0669-0
44. Li, ZD, Li, H, Kang, S, Cui, YG, Zheng, H, Wang, P, et al. The divergent effects of astrocyte ceruloplasmin on learning and memory function in young and old mice. Cell Death Dis (2022) 13(11):1006. doi:10.1038/s41419-022-05459-4
45. Bulters, D, Gaastra, B, Zolnourian, A, Alexander, S, Ren, D, Blackburn, SL, et al. Haemoglobin scavenging in intracranial bleeding: biology and clinical implications. Nat Rev Neurol (2018) 14(7):416–32. doi:10.1038/s41582-018-0020-0
46. Cao, S, Hua, Y, Keep, RF, Chaudhary, N, and Xi, G. Minocycline effects on intracerebral hemorrhage-induced iron overload in aged rats: brain iron quantification with magnetic resonance imaging. Stroke (2018) 49(4):995–1002. doi:10.1161/strokeaha.117.019860
47. Bao, W, Zhou, X, Zhou, L, Wang, F, Yin, X, Lu, Y, et al. Targeting miR-124/Ferroportin signaling ameliorated neuronal cell death through inhibiting apoptosis and ferroptosis in aged intracerebral hemorrhage murine model. Aging cell (2020) 19(11):e13235. doi:10.1111/acel.13235
48. Rivera, AD, Pieropan, F, ChaconDeLaRocha, I, Lecca, D, Abbracchio, MP, Azim, K, et al. Functional genomic analyses highlight a shift in Gpr17-regulated cellular processes in oligodendrocyte progenitor cells and underlying myelin dysregulation in the aged mouse cerebrum. Aging Cell (2021) 20(4):e13335. doi:10.1111/acel.13335
49. Blaho, VA, Galvani, S, Engelbrecht, E, Liu, C, Swendeman, SL, Kono, M, et al. HDL-bound sphingosine-1-phosphate restrains lymphopoiesis and neuroinflammation. Nature (2015) 523(7560):342–6. doi:10.1038/nature14462
50. Zhang, Z, Yu, J, Wang, P, Lin, L, Liu, R, Zeng, R, et al. iTRAQ-based proteomic profiling reveals protein alterations after traumatic brain injury and supports thyroxine as a potential treatment. Mol Brain (2021) 14(1):25. doi:10.1186/s13041-021-00739-0
51. Jing, Y, Yang, D, Fu, Y, Wang, W, Yang, G, Yuan, F, et al. Neuroprotective effects of Serpina3k in traumatic brain injury. Front Neurol (2019) 10:1215. doi:10.3389/fneur.2019.01215
52. Markaki, I, Bergstrom, S, Tsitsi, P, Remnestal, J, Manberg, A, Hertz, E, et al. Cerebrospinal fluid levels of kininogen-1 indicate early cognitive impairment in Parkinson's disease. Mov Disord (2020) 35(11):2101–6. doi:10.1002/mds.28192
53. Mirzaei, M, Pushpitha, K, Deng, L, Chitranshi, N, Gupta, V, Rajput, R, et al. Upregulation of proteolytic pathways and altered protein biosynthesis underlie retinal pathology in a mouse model of Alzheimer's disease. Mol Neurobiol (2019) 56(9):6017–34. doi:10.1007/s12035-019-1479-4
54. Lopez-Otin, C, Blasco, MA, Partridge, L, Serrano, M, and Kroemer, G. Hallmarks of aging: an expanding universe. Cell (2023) 186(2):243–78. doi:10.1016/j.cell.2022.11.001
55. Mattson, MP, and Arumugam, TV. Hallmarks of brain aging: adaptive and pathological modification by metabolic States. Cel Metab (2018) 27(6):1176–99. doi:10.1016/j.cmet.2018.05.011
56. Li, Z, and Rasmussen, LJ. TIP60 in aging and neurodegeneration. Ageing Res Rev (2020) 64:101195. doi:10.1016/j.arr.2020.101195
57. Kato, D, Aoyama, Y, Nishida, K, Takahashi, Y, Sakamoto, T, Takeda, I, et al. Regulation of lipid synthesis in myelin modulates neural activity and is required for motor learning. Glia (2023) 71(11):2591–608. doi:10.1002/glia.24441
58. Ding, J, Ji, J, Rabow, Z, Shen, T, Folz, J, Brydges, CR, et al. A metabolome atlas of the aging mouse brain. Nat Commun (2021) 12(1):6021. doi:10.1038/s41467-021-26310-y
59. Wilkins, HM, and Swerdlow, RH. Mitochondrial links between brain aging and Alzheimer's disease. Transl Neurodegener (2021) 10(1):33. doi:10.1186/s40035-021-00261-2
60. Lautrup, S, Sinclair, DA, Mattson, MP, and Fang, EF. NAD (+) in brain aging and neurodegenerative disorders. Cel Metab (2019) 30(4):630–55. doi:10.1016/j.cmet.2019.09.001
61. Sakamuri, SS, Sure, VN, Kolli, L, Evans, WR, Sperling, JA, Bix, GJ, et al. Aging related impairment of brain microvascular bioenergetics involves oxidative phosphorylation and glycolytic pathways. J Cereb Blood Flow Metab (2022) 42(8):1410–24. doi:10.1177/0271678x211069266
Keywords: proteomics, intracerebral hemorrhage, aging, protein-protein interaction, tandem mass tag
Citation: Li X, Xiao Z, Li P, Yang W, Shen Y, Liu F, Xiong X, Wu Q, Wang P, Dang R, Gui S, Deng L, Manaenko A, Xie P and Li Q (2024) Age-related changes after intracerebral hemorrhage: a comparative proteomics analysis of perihematomal tissue. Exp. Biol. Med. 249:10117. doi: 10.3389/ebm.2024.10117
Received: 01 September 2023; Accepted: 23 February 2024;
Published: 25 March 2024.
Copyright © 2024 Li, Xiao, Li, Yang, Shen, Liu, Xiong, Wu, Wang, Dang, Gui, Deng, Manaenko, Xie and Li. This is an open-access article distributed under the terms of the Creative Commons Attribution License (CC BY). The use, distribution or reproduction in other forums is permitted, provided the original author(s) and the copyright owner(s) are credited and that the original publication in this journal is cited, in accordance with accepted academic practice. No use, distribution or reproduction is permitted which does not comply with these terms.
*Correspondence: Qi Li, cWlsaV9tZEAxMjYuY29t; Peng Xie, eGllcGVuZ0BjcW11LmVkdS5jbg==
†Present address: Anatol Manaenko, Clinical Neuroanatomy Section, Department of Neurology, Ulm University, Ulm, Germany
‡These authors have contributed equally to this work